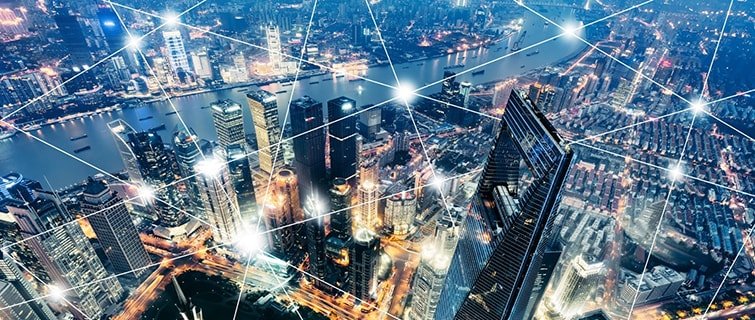
The advice sounds simple; practicing it is another matter.
Asked how young people should prepare for the job market of the future, when the emerging Fourth Industrial Revolution is in full swing, an expert in leadership and organizational development put it this way:
“Be nimble,” said Sukari Pinnock, an instructor in Georgetown University’s graduate program in Master's in Human Resources Management. “People will have to be nimble and be able to turn on a dime.”
Why nimble? Consider the changes predicted for the global workforce: As in the first three industrial revolutions—those that resulted in widespread manufacturing, mass industrialization, and the digital explosion—the fourth, involving the merging of human intelligence with the processes of increasingly “smart” machines, will disrupt our way of living and working in profound ways.
“As whole industries adjust and new ones are born, many occupations will undergo a fundamental transformation,” according to The Future of Jobs, a 2016 report from the World Economic Forum. “Together, technological, socioeconomic, geopolitical, and demographic developments and the interactions between them will generate new categories of jobs and occupations while partly or wholly displacing others.”
Mixing Human and Artificial Intelligence
Winners in this new environment (by job family) include business and financial operations, management, computer and mathematical jobs, architecture and engineering, and sales and related occupations, the report said. The biggest losers will be those jobs that can be outsourced, or—even more cheaply and efficiently—taken over by machines. They include: office and administrative work, manufacturing and production, and construction and extraction.
An oft-repeated estimate is that 65 percent of the jobs that today’s high school and college students will be doing have yet to invented; but that figure has been challenged, and no one can seem to cite the original source. But in the end, it might not matter. In March 2017, experts at an Institute for the Future workshop went even further, predicting that by 2030 the proportion of new jobs will be about 85 percent.
Those jobs will largely involve a mix of human and artificial, or machine, intelligence, said Annie Green, D.Sc., director of the Certificate in Artificial Intelligence Management program at Georgetown’s School of Continuing Studies.
“Students are going to have to be fluent with digital work and the integration of computer and human intelligences, which is not so far-fetched, because that’s where industry is headed,” Green said. “They’re going to have to understand what the computer is doing.”
Improving ‘Soft Skills’
When Pinnock talks about being nimble, she mentions computer literacy, of course, but she also says it’s important to develop the underrated “soft skills” that still separate humans from machines.
“They call them ‘soft skills,’ but they’re not,” Pinnock said. “They’re necessary. They just require different abilities.”
In the future, more white-collar jobs, even those requiring relatively advanced computation, will be taken over by machines. But machines still don’t know how to work in diverse groups (of humans), to brainstorm, to understand the nuances of human emotions, or to recognize one’s implicit biases and use that insight for a common good.
Ironically, these are the kinds of skills that young people may have difficulty acquiring today because they spend so much time with their phones and computers, Pinnock said. As a result, they’ve mastered the abbreviated language of texts and emails and can communicate in this language with others, but they’re not developing more advanced communication skills—the kind they will need for tomorrow’s jobs. And this is something they will have to be taught, she said.
“We have to know how to talk to one another,” Pinnock said. “We have to learn to be with one another, because we’re going to be in the workforce together. In short, we must be both high tech and high touch.”
Glimpsing the Future
About 65 years ago, the huge, unwieldy computers that filled offices and college labs were called “electronic brains” or “giant brains,” writes British linguist W. John Hutchins. And so, in 1954, when the now-famous “Georgetown-IBM Experiment” proved that such machines could translate rudimentary Cyrillic script into English, the newspapers responded—breathlessly—with headlines like: “Electric Brain Translates Russian.”
By today’s standards, it was pretty rudimentary, “a small-scale experiment of just 250 words and six ‘grammar’ rules,” Hutchins writes. Yet it “raised expectations of automatic systems capable of high quality translation in the near future;” and, indeed, Georgetown University’s Leon Dostert, the founder of the University’s Institute of Languages and Linguistics, and one of the project’s leaders, predicted that within three to five years, machine-derived translation “in important functional areas of several languages may well be an accomplished fact.”
As it turned turns out, Dostert’s estimate was off by several decades, but the substance of his prediction was correct.
Today, Benjamin Bengfort teaches the Certificate in Data Science program at Georgetown’s School of Continuing Studies while writing books on textual analysis and doing doctoral work in machine learning and natural language processing.
“I think the best way to describe how far natural language processing has come since the time of the [Hutchins] paper is that we would never think of using hardcoded rules for translation or language processing,” Bengfort said. “We (computer scientists) now think of language as fluid, flexible, and evolving,” and the sheer number of rigid rules that would be required to replace today’s algorithms, which enable computers to ‘learn,’ would “quickly outpace a programmer’s ability to write them—or even hundreds of programmers.”
For the coming years, digital fluency will be critical, Bengfort said. People will have to know basic coding skills because there will simply not be enough computer programmers around to help them in a world surrounded by and infused with machines. Workers in this new environment should have a “security mindset,” for reasons that are apparent today, Bengfort said, and they should develop “the ability to make decisions with data that do not require guessing or intuition.”
We may crave simplicity, but the future will not be simple, says Green.
“People will have to stop being afraid of complexity,” she said. “Nothing, starting out, is simple. In the beginning, it’s going to seem like a lot, but as we move forward it will become more intuitive.”